APPLIED Ai
Neurology
AI models of working memory, visual processing, neuroscience data and computational psychiatry
In Silico Neural Networks
Advances in neuroscience and developments in computer processing have given rise to a new generation of in silico neural networks inspired by the architecture of the brain. These AI systems are now capable of many of the advanced perceptual and cognitive abilities of biological systems, including object recognition and decision making. OptimalAI's clinical neurologists and neuroscientists can support neuroscience research to help transform our understanding of brain functions.
Neuroscience-inspired AI for neuroscience research
Current AI systems are still relatively simplistic in comparison to brain networks. AI models of the cerebral cortex and other brain regions, including limbic and motor control regions are a challenge to creating an AGI system that models the entire brain. Nonetheless, the use of machine learning and neural networks for automated analysis of big data has revolutionized the analysis of behavioral, neuroimaging and objective classification of psychiatric and developmental disorders. OptimalAI's clinical neurologists and neuroscientists are able to utilise neuroscience-inspired AI to help understand how changes in brain networks result in psychopathologies and - potentially - for treatment regimes. In particular, deep learning can be used to model how convolutional layers and recurrent connections in the brain's cerebral cortex can control important functions, including visual processing, memory and motor control.
Classifying psychiatric disorders from neuroimaging data
Psychiatric and developmental brain disorders are primarily identified by a patient’s subjective behavioral symptoms and self-report measures. This can lead to an explanatory gap between phenomenology and neurobiology. OptimalAI can help support AI-based approaches to objective classification of psychiatric disorders. Deep learning approaches for modeling disorders in artificial neural networks can help identify, model, and potentially treat psychiatric and developmental disorders. The multi-layered design of deep neural networks is well suited to learning high-level representations from complex raw data, allowing features to be extracted from neuroimaging data with less parameters than machine learning architectures (Durstewitz et al., 2019, Jang et al., 2017, Koppe et al., 2021, Plis et al., 2014, Schmidhuber, 2015). Several deep neural networks have already been reported to effectively classify brain disorders from neuroimaging data, including schizophrenia (Oh et al., 2020, Sun et al., 2021, Yan et al., 2019, Zeng et al., 2018), autism (Guo et al., 2017, Heinsfeld et al., 2018, Misman et al., 2019, Raj and Masood, 2020), ADHD (Chen, Li, et al., 2019, Chen, Song, and Li, 2019, Dubreuil-Vall et al., 2020), and depression (Chen, Li, et al., 2019, Chen, Song, and Li, 2019, Dubreuil-Vall et al., 2020).
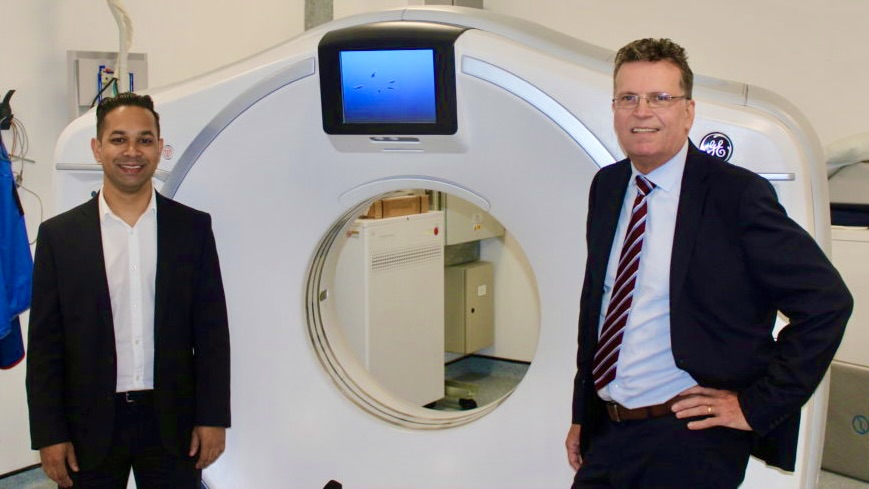
OptimalAI's clinical AI neurologist, Dr Shah Islam (left) and Dr Declan Johnson, receive the 2018 American Society of Neuroradiology Award
Neuroscience-inspired AI for working memory
OptimalAI can help neuroscientists and clinicians to better understand information processing and memory. Spiking recurrent neural networks (SRNN) models are just one avenue for OptimalAI to support research into the working memory mechanisms of the brain. In many ways, the relationship between neuroscience and AI research has come full circle, with recent SRNN models potentially informing us about brain mechanisms underlying working memory. SRNN models of working memory can help recreate the recurrent connections between neurons within the brain’s cerebral cortex and perform a wide variety of cognitive tasks that require working memory. Spiking networks can provide improved biological plausibility over recurrent neural networks (RNN), offering advantages for modeling and potentially informing how working memory may be controlled in the brain (Diehl et al., 2016, Han et al., 2016, Pfeiffer and Pfeil, 2018, Taherkhani et al., 2020). In a 2021 study, an SRNN trained on a working memory task showed similar temporal properties to single neurons in the primate prefrontal cortex. The model provided evidence of a disinhibitory microcircuit that acts as a critical component for long neuronal timescales that have previously been implicated in working memory maintenance in real and simulated networks.
Decoding the brain's visual system
OptimalAI can help create artificial systems to reverse engineer the brain’s ventral visual stream to decode and interpret human visual processing, as well as performing complex visual intelligence skills including image recognition, motion detection and object tracking. Considering the complexity of the brain’s visual system, including the existence of multiple cell types (Gonchar et al., 2008, Pfeffer et al., 2013) that are modulated by multiple neurotransmitters (Azimi et al., 2020, Noudoost and Moore, 2011), it is unlikely that artificial neural networks can currently fully model the myriad of processes contributing to biological visual processing. However, in an effort to understand and measure human visual perception, machine learning models - including support-vector networks - can been trained to decode stimulus-induced fMRI activity patterns in the human V1 cortical area can visually reconstruct the local contrast of presented and internal mental images (Kamitani and Tong, 2005, Miyawaki et al., 2008). Also, by adding deep generator network (DGN) to a very deep convolutional neural network (CNN) image reconstruction method, allowing CNN hierarchical processing layers to be fully utilised in a manner similar to that of the human visual system, has recently been demonstrated to improve the quality of visual reconstructions of perceived or mental images compared with the same CNN without the DGN (Shen, Horikawa, Majima, & Kamitani, 2019).
AI in neuro-oncology radiology
AI in neuro-oncology radiology have been shown to predict patients' outcomes more accurately than conventional parameters. In particularly deep learning, has demonstrated remarkable progress in image-recognition tasks. OptimalAI's clinical AI neurologists are world leaders in neuro-oncology radiology and have supported organizations including IBM and the NHS in the training of AI models to automatically detect and localize brain tumors from scans.