RESEARCH AREAS
Practices for responsibility
AI as a force for good
We are driven by the belief artificial intelligence (AI) can be a transformative force for good. However, we also recognize the responsibility that comes with this potential. By adhering to a human-centered approach in fundamental AI research and real-world applications, we aim to create AI systems - and eventually Artificial General Intelligence (AGI) systems - that enhance the human experience, empower individuals, and contribute positively to humanity. OptimalAI's Responsibility in AI is a set of tangible practices that underpin our research, development and application of AI.
Practices
Ethical Considerations
Safety First
Human-centered AI should be designed with robust safety mechanisms to prevent unintended consequences and ensure that humans have control over the technology. This involves addressing issues related to the system's behavior, decision-making, and potential risks.
Collaboration
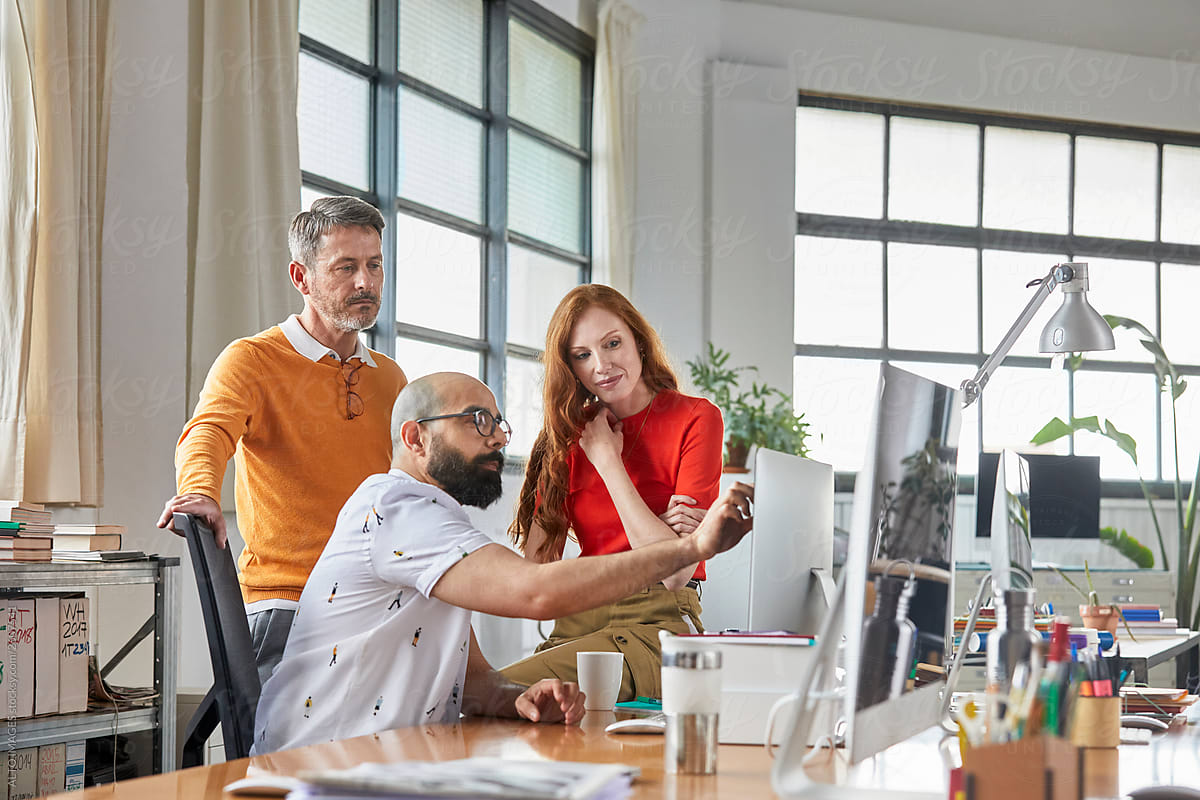
Fairness
Equity
Algorithmic Transparency & Explainability
Making AI transparent and explainable to users is essential. Users should understand how and why the AI system reached its conclusion and have confidence in its decision-making processes. We strive to make the decision-making processes of all of our AI systems as transparent and comprehensible as possible and empower users to override or enhance that conclusion.
Interpretability
Explainability
Privacy
Data Protection
OptimalAI places a strong emphasis on data protection. We employ robust measures to ensure the secure handling and storage of user data, respecting privacy rights and regulations.
Consent and Control
Safety and Security
Robustness
Deployment Standards
Uncertainty and Traffic-Aware Active Learning
The traditional method of training a semantic parser - a tool used to understand natural language - involves collecting and annotating large amounts of data, which is expensive, time-consuming and raises privacy concerns as customer data needs to be handled by human annotators. Uncertainty and Traffic-Aware Active Learning is a new method that selects utterances (or spoken phrases) for annotation based on how often they appear in customer interactions and how confident the model is in understanding them. The technique proved significantly better than previous methods when tested on both an internal customer dataset and the Facebook Task Oriented Parsing (TOP) dataset. Remarkably, it achieved the same accuracy as the traditional random sampling method, but used 2,000 fewer annotations, thereby proving more efficient.